Podcast Drop: A Conversation with Jake Graham, CEO of Bobsled
Why cross-cloud data sharing is critical in helping power the AI Era
Please subscribe, and share the love with your friends and colleagues who want to stay up to date on the latest in artificial intelligence and generative apps!🙏🤖
Last month we had the pleasure of sitting down with Jake Graham, CEO and Co-Founder of Bobsled, a cross-cloud data-sharing platform. In a recent post we also highlighted the increasing importance of data labeling in the era of AI. While data labeling is a critical step, another equally important step is data sharing.
To continue making progress in this AI era, data has to be able to move between the systems and organizations where it’s generated.
Prior to co-founding Bobsled, Jake spent ~18 months building the strategy and plan to create an Azure Data Exchange and an Azure data ecosystem to make data significantly easier to share across Azure consumers. Jake believes that data sharing will be how data is accessed for modern analytics and ML pipelines. But in order to make that happen, the data producers needed a way to interact with all of these systems without having to do it all themselves. That’s fundamentally what Bobsled is.
Jake shared several key learnings on how Bobsled is making data accessible and available in any cloud or data platform. You can listen to the full podcast on Apple or Spotify, or read the transcribed version here. Below we highlight some of the key takeaways, but encourage you to listen to the full podcast.
Let’s dive in!
What does Bobsled Do?
Bobsled is a data-sharing platform that allows our customers to make their data accessible and available in any cloud or data platform where their customers work without having to manage any of the infrastructure accounts, pipelines, or permissions themselves. You grant Bobsled read access to wherever you store your data, whether in files in an S3 bucket or indirectly within Databricks in BigQuery, Snowflake, etc. You reach out to our API to say, “This data needs to be consumed by this individual in this cloud, this data platform, and be updated in this way.”…It allows them to move from putting all the work on their consumer to making it easy without suddenly having to manage an infrastructure footprint in every platform where their customers work.
The volume of data used for analytics, data science, and machine learning has grown a couple of orders of magnitude over the last decade, but the actual mechanisms for that data to be accessed are almost exclusively the same as ten and even 20 years ago. The overwhelming majority of data that’s used to drive any form of data pipeline is either pulled out of an API or an SFTP server. That doesn’t make sense in a world where so much of the value being generated by modern enterprises is in data and in which you need that data to be consumed by others, whether in your organization or others, to extract that value. We needed to see the cloud-native data exchange mechanism take off.
That sharing mechanism was pioneering, and every other major platform followed it. The problem is that it puts a significant infrastructure burden on the actual producer. We want to move away from a world in which every data consumer has to ETL the data out of whatever platform it’s in. The issue you get with that is that sharing protocols aren’t connectors. It’s not just taking the traditional model of we’re going to toss data over the wall. They provide a better consumer access experience because you have to bring the data to where it will be consumed. You have to structure it in a way that is ready for analytics and then grant access to it.
How Is Data Sharing Relevant for Intelligent Applications?:
One of the things that people are starting to realize is that often, in any application, a lot of the value it provides is actually in the data generated by running that application. There’s an enormous amount that you can do in that workflow to use that data to improve it and continue to automate it. Another thing we’ve learned about data over the past decade is that it becomes valuable when it’s blended with other data sets. Within data, almost always, the whole is greater than the sum of its parts. When you realize that if you want to think intelligently and predict, and I think even more if you want to do that in an automated fashion using LLMs, you have to be able to bring in the data that represents different aspects of a problem, and that is never sitting in one system.
We saw a push led by Salesforce around the Salesforce Data Cloud. Well, if we can get everyone to bring all of their data into our application, we can solve all the problems. And the answer is no, you can’t. You might be able to answer many questions, but in reality, data is being generated across this enterprise and its partners and other vendors. It needs to flow into the systems where it’s going to generate insight. I fundamentally believe that data sharing will be the mechanism to do that.
How I think this shift enables the move toward the age of AI is we’re going to allow every company to create data products and to have them be accessible wherever they need to work without, again, having to manage an infrastructure footprint and have an army of people who understand how does clustering work differently in Databricks versus Snowflake. Bobsled is going to be a lot of the plumbing for how the world becomes AI-driven.
Where the Modern Data Stack is Today?
My general feeling about the modern data stack is it’s no longer a valuable term because it won. There was a time when the modern data stack described a few companies and categories that were bringing analytical infrastructure into becoming cloud-native.
The modern data stack is just now a key part of technology. We’re moving from a purely software-centric technology market to a data-centric one. That’s the idea for me of intelligent applications, or if you want to call it the age of AI. The software is incredibly important, but it needs the data. It’s no longer enough to build for a very specific set of users in a very specific category. We now have a much larger field to play in, but also, it’s a much more competitive field.
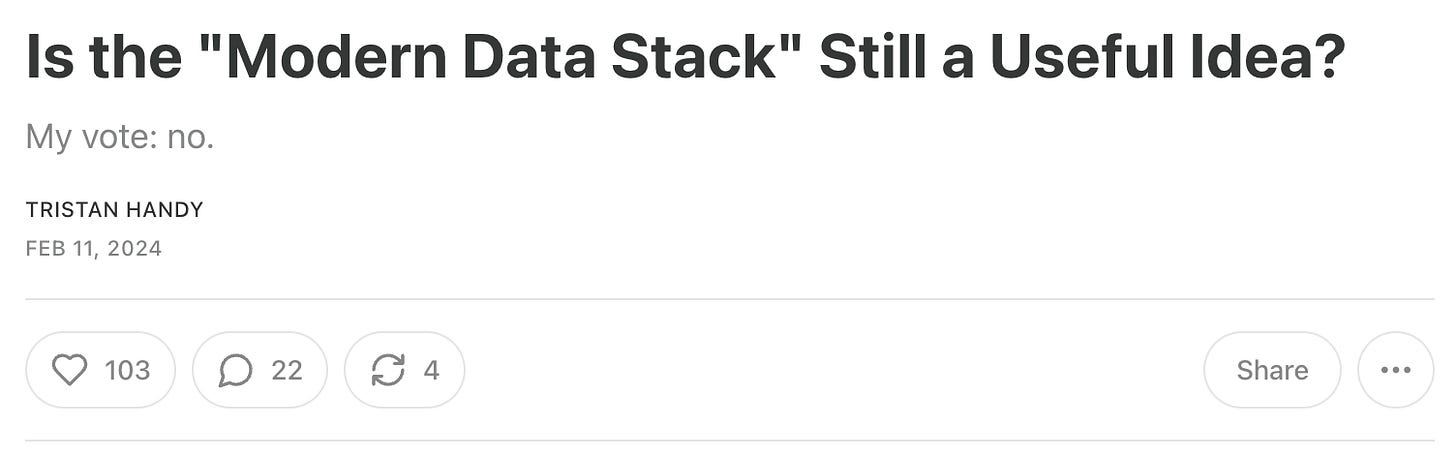
Why You Can’t “PLG” the Enterprise:
Holistically, as an industry, we’ve lost respect for the enterprise sales part of enterprise software. The pendulum has swung a little bit too far toward the product should sell itself in some ways that’s for really positive and great reasons. It has pushed us to think about product design and user experience. A lot of it has been pushed by individuals within organizations being much more empowered to adopt technology. There are hundreds of millions or billions of dollars in truly product-led growth revenue happening every day. I’m not saying that’s not real, but it doesn’t consider how large enterprises make decisions around technology.
If you think of a few things, often, your buyer and your user are not the same person. Generally, if you’re building something that’s of strategic value and is looking for, you’re not starting small; you need to be attached to a strategic initiative in which there are multiple decision-makers, not just the person who will be using your product. Creating not just a sales motion that allows you to get in front of those people, understand their requirements and goals, navigate their organization, and transfer your excitement about your product to them. That’s a big part of what people have missed: the art, craft, and need for actual enterprise selling.
Every company, but really every startup, you live and die based on your feedback loop. Focus on a problem, not your solution; ship quickly, get feedback, and iterate. That is awesome in a PLG world where the cost of getting that feedback is incredibly low. It’s challenging in the enterprise space because there is a gap between your buyer and your user. It’s often easier to get time with executives and the users who are going to implement. You’re not getting perfect information there. If you are building something entirely net new, like there is no direct equivalent to Bobsled, even your user will think they’re going to understand how they’re going to use their product. And it’s going to be somewhat wrong once you actually get into production.
Advice for Partnering with Cloud Providers?
My advice for the early stage would be to focus on partnering with individuals at hyperscalers. So all of these companies have effective machines that move billions of dollars in revenue for partners, and almost none of those billions of dollars in revenue come from early-stage startups.
Anything that you can do to focus on where you are driving value they care about is similar to enterprise sales in the same way. If you’re trying to sell something, you must attach it to a strategic initiative that people care about.
Thanks to Jake for the great conversation. And btw, Bobsled is hiring…check them out here!
We hope you enjoyed this edition of Aspiring for Intelligence, and we will see you again in two weeks! This is a quickly evolving category, and we welcome any and all feedback around the viewpoints and theses expressed in this newsletter (as well as what you would like us to cover in future writeups). And it goes without saying but if you are building the next great intelligent application and want to chat, drop us a line!