Five Predictions for AI in 2025
Our third annual tradition of making predictions in a wildly unpredictable market
Please subscribe, and share the love with your friends and colleagues who want to stay up to date on the latest in artificial intelligence and generative apps!🙏🤖
2024 was another big year in AI. Giants like OpenAI, Anthropic, and Databricks (which just came off a record-breaking $10B fundraise) each soared to new heights while emerging AI startups like Sierra, Cursor, Read, and others made their presence known. While there were occasional disappointments (anybody remember the Rabbit R1?), the pace of innovation in the space continued to charge full steam ahead.
So what is 2025 going to bring? In a world where virtually every company claims to be “AI-powered”, it’s more difficult than ever to parse out what is “real” or not. Is that going to stop us from making predictions based on (some) data and (mostly) our intuitions?
Absolutely not.
Continuing our annual year-end tradition of making predictions in an inherently unpredictable market, we believe these five themes will have a major impact in AI in 2025.
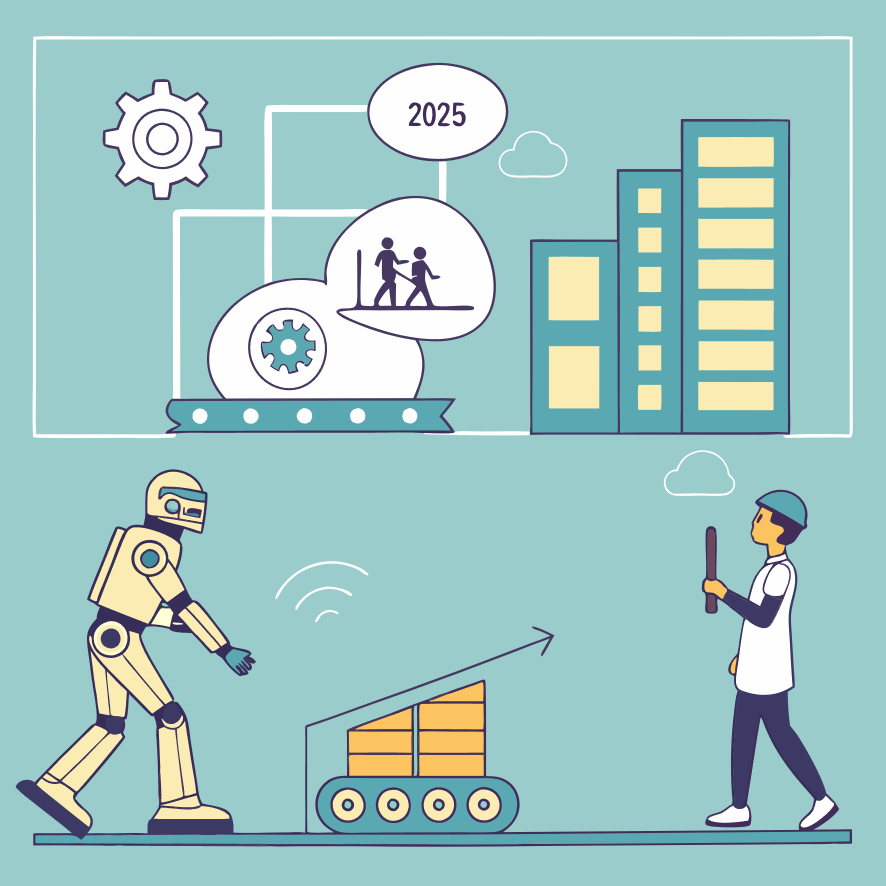
1) Models shift from Pre-Training to Post-Training
Over the past several years, large language models have improved largely based on the amount of data they are fed and trained on. The more data the model is fed during “pre-training” (i.e., the process of training a model on a large dataset to learn general features and representations before finetuning it), the better it will perform. For example, GPT-3.5 was trained on 175B parameters, while GPT-4 was rumored to have 1T+ parameters, leading to “better contextual understanding and response coherence.” The raw amount of data, largely derived from scraping the Internet, fueled the massive growth in pre-training.
Now, we are starting to reach the outer limits of what pre-training can do.
At NeurIPS this year, Ilya Sutskever captured this dynamic well when he declared that “Pre-training as we know it will unquestionably end.”
"Data is not growing because we have but one internet. You could even say that data is the fossil fuel of AI. It was created somehow, and now we use it, and we've achieved peak data, and there will be no more — we have to deal with the data that we have."
In other words, we are shifting from PRE-TRAINING to POST-TRAINING; moving away from simply feeding models as much data as it can handle, towards determining how to make models smarter and more efficient once the data has already been ingested.
Several exciting developments in post-training are emerging, including:
Supervised finetuning: This process refines a pre-trained model with labeled data, providing explicit examples of input-output pairs to guide the learning process. Applied correctly it can make training more efficient and cost-effective.
Two-phase post-training: Some models, like Qwen 2.5, employ a two-phase approach, starting with supervised instruction fine-tuning followed by further optimization.
Long-context training: Increases the context length at the end phase of pre-training, allowing for better handling of lengthy data.
Direct preference optimization: Uses a binary cross-entropy objective to steer models toward producing the types of responses that people “actually want to see.”, eliminating the need for building and tweaking a separate reward model.
If you’re interested in reading more about new paradigms in post-training, check out Sebastian Raschka’s great blog post here.
Another technique we expect to hear a lot more about in 2025 is “test-time compute”…
2) Test-Time Compute Becomes the New Paradigm
One key trend we anticipate for 2025 is the rise of test-time compute. This concept involves allocating additional processing power during inference (execution) to improve model performance. In practice, this means models can generate multiple solutions, evaluate them systematically, and select the best one - a significant departure from the status quo. We believe this shift will influence everything from user interactions to the increasing relevance of inference-optimized hardware like specialized chips.
Rather than relying on large pre-training budgets alone, test-time compute leverages dynamic inference strategies that allow models to "think longer" when faced with harder tasks. Methods for achieving test-time compute include reward modeling, self-verification, search methods, best-of-n sampling, star algorithms, verifiers, and Monte Carlo tree search. Among these, two primary strategies stand out:
Self-Refinement: Models iteratively refine their outputs by identifying and correcting errors
Search Against a Verifier: Models generate multiple candidate answers and use a verification system to select the most accurate result
So what are the implications we expect to take place in 2025?
Enhanced Performance in Math, Finance, and Engineering: Historically, these fields have been challenging for LLMs due to their inability to verify outputs or handle complex, multi-step reasoning. Tasks in these domains require iterative refinement and validation. We imagine there will be applications in 2025 that emerge around the ability to solve math problems, detect fraud, or perform advanced spreadsheet analysis. These use cases, which demand precision and validation, could become more prevalent.
Advances in Healthcare, Medicine, and Scientific Research: Breakthrough in fields requiring hypothesis testing and iterative refinement, such as physics, chemistry, and biology. For example, in drug discovery or clinical trials, models could simulate and refine molecular designs or test hypotheses with greater accuracy.
Improved Multi-Modal Reasoning: By simulating human-like iterative reasoning, LLMs could tackle complex problems by breaking them into smaller parts, validating each step, and integrating insights across modalities (e.g., text, images, and numerical data).
Test-time compute allows such systems to operate with higher accuracy, adaptability, and context awareness. These capabilities are vital for tasks that go beyond summarization and require deeper "thinking," such as navigation, robotics, or complex problem-solving. Importantly, these systems would focus on leveraging existing knowledge rather than generating entirely new information.
3) Reasoning and Inference (Chips) Take Center Stage
2025 will also mark an overall shift from TRAINING to INFERENCE as reasoning becomes more important, creating heightened demand for inference-based chips.
As AI models grow in size and complexity, they require more computational power for inference tasks. For example, OpenAI's o1 model demonstrates advanced reasoning abilities, producing long internal chains of thought and breaking down complex problems into simpler steps before responding.
A recent article from The Atlantic, provocatively titled “The GPT Era Is Already Ending”, explores this shift more deeply:
Mark Chen, then OpenAI’s vice president of research, told me a few days later that o1 is fundamentally different from the standard ChatGPT because it can “reason,” a hallmark of human intelligence. Shortly thereafter, Altman pronounced “the dawn of the Intelligence Age,” in which AI helps humankind fix the climate and colonize space. As of yesterday afternoon, the start-up has released the first complete version of o1, with fully fledged reasoning powers, to the public.
This trend towards more sophisticated reasoning in AI models will drive the need for specialized chips optimized for these tasks.
NVIDIA, the largest player in the AI chip market (and arguably the biggest winner of the AI era so far) has mined gold for the past two years thanks to its training-focused GPU chips like the H100s and A100s. Unlike training, inference chips are optimized for speed and efficiency, executing pre-trained models to make real-time decisions based on new data. Additionally, inference chips must balance computational power with energy efficiency, enabling their integration into power-sensitive devices like smartphones and IoT products.
We expect 2025 to be a good year for specialized inference chip providers like Cerebras, Groq, and SambaNova. Of course NVIDIA won’t go away anytime soon, but it will be fun to see whether the balance of power starts to shift in this new paradigm.
4) Agent Are (Finally) Unleashed
Last year, we anticipated a proliferation of agents across intelligent applications. While the concept gained significant traction, with many companies exploring ways to embed agents into workflows, the execution largely remained conceptual. Few companies have fully deployed agents in meaningful, scalable ways. However, we believe 2025 will be the inflection point where agentic frameworks and infrastructure mature, enabling semi-autonomous agents to thrive, especially for internal use cases.
At the application layer, we believe agents will proliferate across:
Consumer agents: New paradigm shift specifically around the UI/UX. We expect to see more voice-first experiences where conversational agents handle tasks seamlessly. We are also looking out for more personal assistants as we move from simple task management to personalized recommendations for daily activities (e.g., booking a trip, planning an event, fitness plans, coaching, learning).
Horizontal agents: We’re most excited about agents powering functional workflows and offering general-purpose functionality across sales, search & knowledge, design and creativity, software development, and security. Core use cases will emerge around document extraction and processing, summarization, and task execution.
Vertical agents: We’re keeping an eye out on agents tailored to specific domains in healthcare, legal, insurance and finance.
At the infrastructure layer, we believe these key advancements will help unlock agentic applications:
Reasoning becomes more advanced: Agents will develop the ability to plan, prioritize, and self-validate. One thing we’ll be watching is how agents will be able to prioritize actions based on real-time inputs and adjust dynamically as information emerges.
API orchestration & vision-based frameworks: Unified frameworks will enable agents to seamlessly integrate with APIs, process multimodal data, and leverage computer vision for tasks like computer use, medical imaging, and more.
Security & auth: Stronger safeguards, such as role-based access control, behavior monitoring, and zero-trust models, will ensure agents operate securely while protecting sensitive data.
5) Consolidation of Early GenAI Companies and New Biz Models Emerge
Throughout 2022 and early 2023, we saw a rush of funding towards GenAI startups of all flavors - we wrote more here. Many of these companies were deemed "wrappers around GPT models," showcasing flashy demo features that helped secure sizable pre-seed or seed rounds, though not necessarily built with a strong moat. However, with most startups raising only about two years of runway, those funded in 2022 and 2023 but struggling to achieve PMF will face pressure to find an exit or risk shutting down. While 2024 has already seen a rise in M&A activity, we expect 2025 to mark the beginning of significant early generative AI acquisitions, with two primary acquisition strategies emerging.
Acquihires for tech and talent: Companies like OpenAI, Anthropic, Meta, Databricks, Salesforce, etc. are sitting on loads of capital and want to bring on the best talent to continue their dominance. They are already willing to pay multi-million dollar compensation packages to the best AI talent, and we expect more acquihires will occur as a result.
Gen-enhanced pre-IPO companies: We predict that later-stage, gen-enhanced pre-IPO companies will acquire gen-native startups to craft a more compelling AI narrative for investors ahead of their IPOs. These acquisitions will help strengthen their positioning and showcase a cohesive AI strategy to the market.
We also expect to see more companies testing different business models. The two business models we’re keeping an eye on are:
Outcome-based pricing models: Many software companies have tried outcome based pricing models in the past but have failed because it’s difficult to accurately attribute value towards a specific piece of software. As we start to see more agentic workflows capable of completing specific tasks (e.g., “answer this ticket”) it may make this pricing model more feasible. Companies like Sierra are already testing this out.
Service as software: Startups are looking to become more efficient and outsource traditional consultancy and SI work to an “AI-native” company. Under this model, a GenAI company delivers outcomes by combining human expertise with AI to optimize workflows. Companies like QA Wolf and SeekOut are pioneering these hybrid approaches, blending human involvement with AI-driven processes. We expect this model to gain traction in verticals, such as software development, customer support, ITSM, and security where the combination of AI and human expertise can deliver scalable, high-quality results.
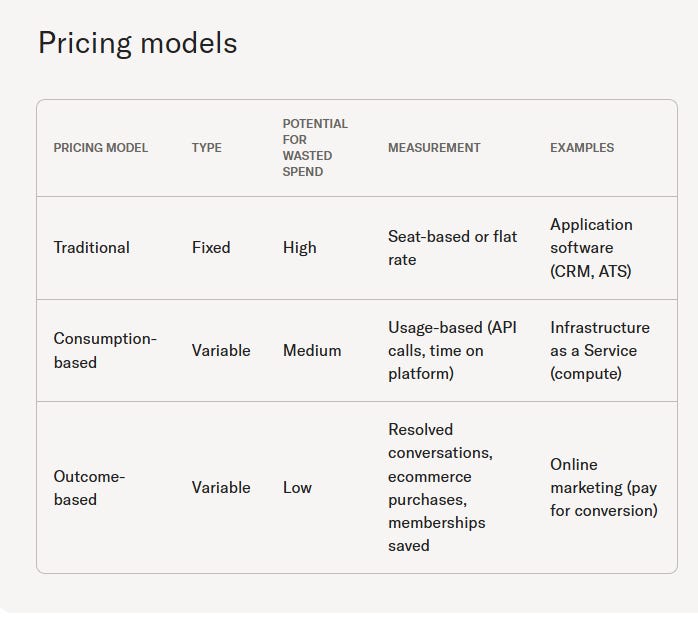
In Conclusion…
The GenAI continues to evolve rapidly, and 2025 is shaping up to be another pivotal and impactful year. While we don’t expect to get to AGI just yet, we’re likely not too far away…
In addition to the five areas we highlighted above, we’re excited to see advancements in other fields like open-source models, smaller language models, edge deployments, and quantum computing (Willow from Google is pretty cool). If we’re lucky we’ll also see the potential for GenAI to accelerate breakthroughs in biotech, particularly in drug discovery and computational biology.
In conjunction, we hope to see more progress in regulatory frameworks and compliance discussions. Innovation thrives when paired with accountability, and 2025 must be a year where we balance pushing boundaries with safeguarding societal impact.
As we look to 2025, we are excited and inspired by what’s to come. Thanks for continuing to support our work and see you in the new year!
Thank you for all you guys do. Love reading these every week. Merry Christmas and Happy New Year!