Happy first few weeks of the fall! Thank you to our now 1.5K+ subscribers, we appreciate all your support! Please continue to share with colleagues, friends, or anyone you think would enjoy staying current on the latest trends in AI :)
An interesting debate was sparked last week on the topic of whether or not we have an oversupply of AI infrastructure. David Cahn at Sequoia penned a blog post arguing that based on current GPU production and data center costs, the AI market has a “$125B+ hole that needs to be filled for each year of CapEx at today’s levels”, certainly an ambitious target in any industry. In an X thread, a16z’s Guido Appenzeller countered that David’s analysis i) blends together one-time capex and annual opex costs; ii) overstates the energy costs required to build GPUs, and most importantly iii) underestimates the long-term demand for AI infrastructure.
Beyond the good old-fashioned VC vs. VC intellectual sparring, the exchange helped bring forth an important examination of the supply and demand imbalance we are witnessing in AI.
So what’s really going on here? In our analysis, we believe there is some truth to both what David and Guido are saying. There is certainly an eye-popping amount of AI capex being built right now, but at the same time, like many prior technological waves harkening back to railways, demand is non-deterministic and tends to follow supply.
In other words: if you build it, will they come?
Let’s read on…
Capex and Infrastructure are often a Prerequisite for Innovation
With large-scale paradigm shifts like what we are experiencing with AI, it is instructive to look back at other times in history when a capex-heavy technology laid the groundwork for vast economic gains.
The Transcontinental Railroad in the 1860s
The construction of the transcontinental railroad in the United States in the late 18th century was a massive infrastructure project, with some estimates pegging the cost at ~$100M at the time, or ~$2.3B in today’s dollars, a previously unimaginable figure. It was a gigantic undertaking and took six years and thousands of men to complete. However, the railroad had a profound impact on the U.S. economy, opening up new markets for goods and services, and stimulating GDP growth. By 1880, 11 years after the railroad’s completion, $50M worth of freight traveled by the rails each year.
But the vast majority of the railroad’s impact came not from the obvious commercial uses, but the broader impact on society. The transcontinental railroad led to a doubling of the population in the American West between 1870-1880, a tripling in the number of businesses (including the first mail-order catalog company), and even the creation of time zones in 1883. None of this would have been possible without the initial infrastructure investment whose cost was primarily borne by two competitors: Union Pacific and the Central Pacific.
Electrification of America in the 1930s
A few decades later, efforts to bring electricity to the majority of the US began. While Edison and Westinghouse had introduced practical electric power systems as early as the 1880s, by 1931 still only 10% of rural America (which comprised the majority of the nation) had electricity. To rectify this problem, the US government partnered with private businesses to construct power plants, lay transmission lines, and create distribution networks. Some estimates put the total cost of electrification at over $150B in today’s dollars.
However, the investment in electricity infrastructure paid off handsomely. It’s hard to think of a world prior to electricity. While at the time most efforts to electrify households revolved primarily around one key application (lightbulbs), the increase in the supply of electricity also catalyzed the demand for modern applications like TVs, air conditioners, refrigerators, toasters, irons, and so much more. In this case, the investments into increasing the supply of a scarce resource were significantly outstripped by the increase in demand for that resource.
The Telecom Boom of the 1990s
Jumping forward to the age of tech, the modern Internet era can partially trace its roots to the Telecommunications Act of 1996, a landmark piece of legislation signed by President Clinton that ushered in private competition in the broadcasting and telecommunications markets. In the subsequent five years, telecom companies “poured more than $500 billion into laying fiber optic cable, adding new switches, and building wireless networks.” This massive infrastructure spend had the short-term effect of creating an unsustainable growth in overvalued businesses, leading to the Dot-Com crash of 2000. As late as December 2002, an analyst at Brookings quipped that while “broadband satisfies consumers’ quest for speed…so far no ‘killer application’ has emerged to make it a ‘must have’ service. In particular, the much ballyhooed video-on-demand has yet to arrive.”
Twenty years later, its clear that many “killer applications” emerged: Google, Amazon, Uber, Airbnb, Doordash, and thousands of other consumer applications generate billions in revenue thanks to the infrastructure underpinning the modern Internet. In 2020, the internet economy was estimated to contribute nearly 10% of the $21T in US GDP, dwarfing the $500B of infrastructure spend that was assumed to be “lost” after the Dot-Com crash.
Today, it is unfathomable to think of what the world would look like today without the Internet. And of course, the “much ballyooed video-on-demand” finally arrived :)
The Demand Side: Products and Consumption
Does GPU capacity generally get filled? Is there enough demand in the market, or will GPUs be going idle? Looking at the prior mini-cycle of crypto, Nvidia was building GPUs to fulfill that demand. Here, you could make an argument that while crypto didn’t lead to large productivity gains for society, the demand was filled.
In the AI case, time will tell. We know that not all consumption of GPUs will show up directly as “AI revenue” and we know there are both current use cases and future use cases. Today, we are witnessing the demand for GPUs ranging from startups to public companies, Non-Tech to BigTech, as they all race to both build new AI applications as well as fold AI into existing products. We also know that demand may continue to skyrocket so long as the AI hype continues.
Today, there are not enough high-quality, high-performance chips to satisfy demand. In particular, companies are looking for H100s (and A100s for inference). H100s are the fastest and most efficient GPU in the market for training and inference. This demand is primarily being driven by a few key factors:
Companies building their own LLMs from scratch (e.g., OpenAI, Inflection, Anthropic, CharacterAI, etc.)
Training & Inference: Training is the process of teaching the ML model to make accurate predictions; whereas inference is the deployment of the trained model to make predictions on new, unseen data. Key components include memory bandwidth, caches and cache latencies, compute performance, interconnect speed, and more.
Large private clouds (e.g., Lambda, CoreWeave are starting to see more demand from Enterprises who would typically be defaulting to larger cloud providers)
Enhanced AI & ML capabilities (e.g., Chinese companies like ByteDance, TikTok, Baidu and Tencent have been buying GPUs for enhanced performance of AI algorithms for content recommendation, personalization and other AI features)
Data processing (e.g., Financial institutions like JPM, Two Sigma, and Citadel have been investing in GPUs for increased data processing, data analytics, and processing of large data sets).
Today, it feels like we continue to have a very high level of demand for these GPUs as all AI companies need to train and run inference on for the best model performance. While Nvidia is the primary player in the hardware ecosystem for AI computing, competitors like AMD, Intel, and newer startups like Cerebras Systems aren’t going to sit back and just let them win uncontested.
So, Where Do The Lines Intersect?
It may take years before demand and supply for GPUs intersect and we have equilibrium. But from other transformative technological shifts in history, we know that large upfront capex investments tend to lead to a reduction in costs for consumers, thus spurring more demand:
In the 1860s, the cost of a six-month stagecoach trip across the US was $1,000 (~$20K today). After the Transcontinental Railroad was built, the trip took 5 days and cost $150 (an 85% drop in price). Not surprisingly, the demand for travel skyrocketed.
From an Economist article: “In real terms, the price of electricity between 1900 and 2000 dropped nearly 100%, the magazine found.” Over the same period, the usage of electricity, and the creation of new electronic products, dramatically increased.
As for AI and the GPU demand, while we know Nvidia competitors are challenging the incumbents with bold and innovative designs, there are many challenges faced by hardware designers to keep up with AI progress on the software side.
Training Costs → Today, training a model is very costly (GPT-4 for example is reported to have cost over $100M). But as more advancements and improvements happen (i.e., algorithmic improvements and newly available hardware) costs will come down which will unlock other parts of the market to come in.
GPUs aren’t consumed immediately → Might we have an oversupply of GPUs at large tech companies? Some companies may realize they have over-committed their need for GPUs. If the market cools around AI use cases, prices will also come down. Perhaps we may see new use cases materialize?
Open-source models play a role → Open-source may be more widely used resulting in less need for specialized LLMs.
AI Hype and AI FOMO die down → Some of the promises of AI may not take shape as quickly as expected and products may not work out as well as intended, thus the need for more GPUs will decrease.
Conclusion
We think David and Guido both bring up good points: there is a massive buildup of capex and infrastructure associated with AI today, particularly around GPUs. At the same time, the costs aren’t likely as high as we think, and there already exists a significant demand for that supply (and revenue will show up in many different areas).
In our view, history has shown that in transformative technological cycles, infrastructure costs always run high and precede demand. But that demand comes quickly, and in forms never previously imagined.
At some point, as we’ve seen previously with railroads, electricity, phones, and the Internet, the supply and demand curves will intersect, and when they do, another phase of economic growth begins.
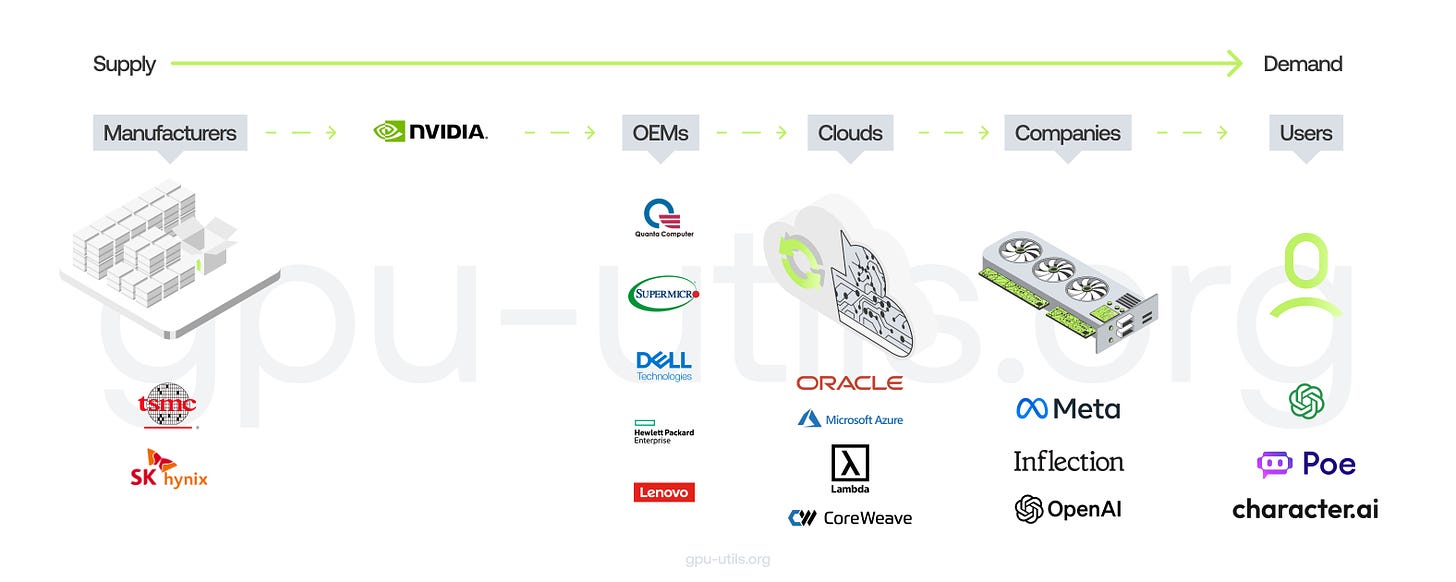
If you want to read more about this hot debate of GPU shortages, this is one of the more detailed articles we recommend reading here
Funding News
Below we highlight select private funding announcements across the Intelligent Applications sector. These deals include private Intelligent Application companies who have raised in the last two weeks, are HQ’d in the U.S. or Canada, and have raised a Seed - Series E round.
New Deal Announcements - 09/15/2023 - 09/28/2023:
We hope you enjoyed this edition of Aspiring for Intelligence, and we will see you again in two weeks! This is a quickly evolving category, and we welcome any and all feedback around the viewpoints and theses expressed in this newsletter (as well as what you would like us to cover in future writeups). And it goes without saying but if you are building the next great intelligent application and want to chat, drop us a line!
One of the best AI/LLM primers/perspectives I've read! Thanks for sharing